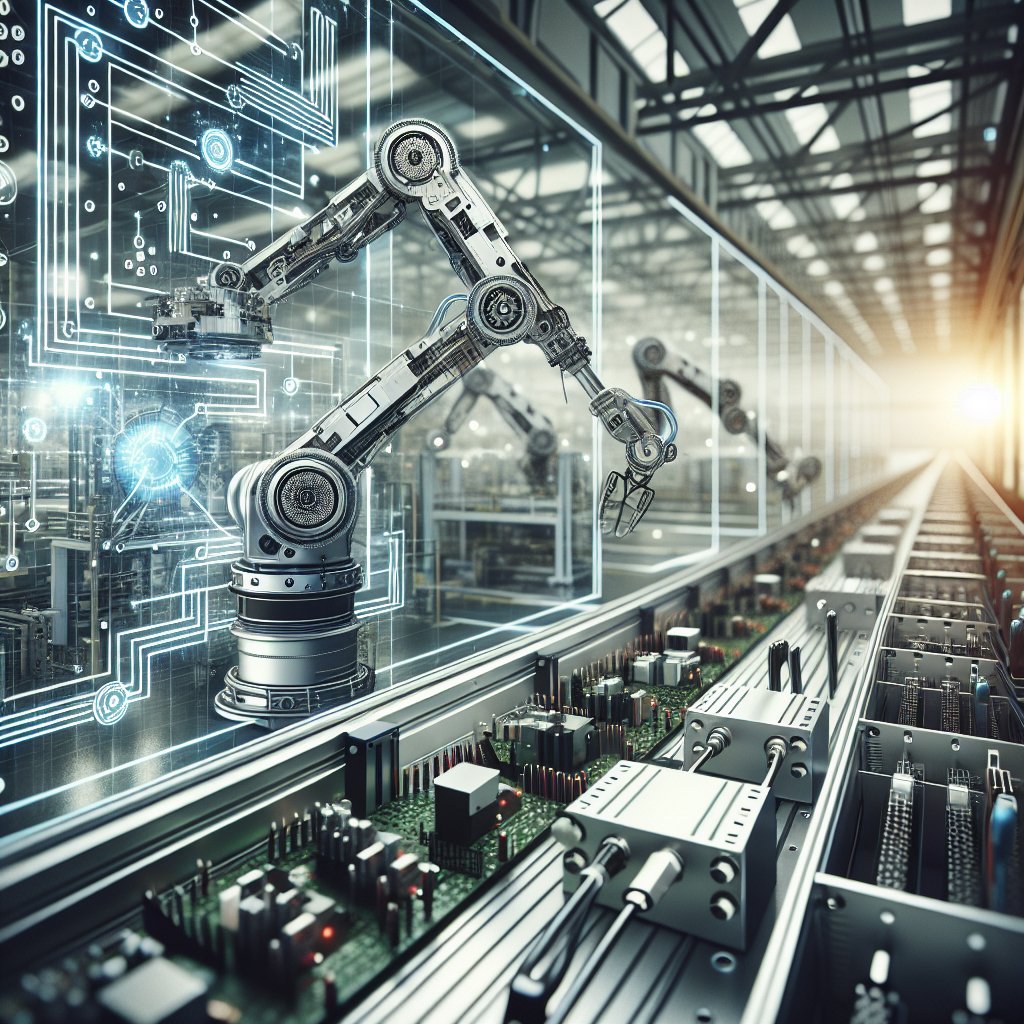
Machine learning has emerged as a transformative force in the realm of industrial control systems, offering unprecedented opportunities for optimization, efficiency, and innovation. As industries increasingly adopt smart technologies, the integration of machine learning into control systems is becoming a pivotal factor in maintaining competitive advantage. This article delves into the various applications of machine learning in industrial control systems and explores the challenges that accompany its implementation.
Applications of Machine Learning in Industrial Control Systems
Machine learning is revolutionizing industrial control systems by enabling more intelligent and adaptive processes. One of the primary applications is predictive maintenance, where machine learning algorithms analyze data from sensors and equipment to predict failures before they occur. This proactive approach minimizes downtime and reduces maintenance costs, leading to significant improvements in operational efficiency.
Another critical application is process optimization. Machine learning models can analyze vast amounts of data to identify patterns and correlations that are not immediately apparent to human operators. By leveraging these insights, industries can optimize production processes, enhance product quality, and reduce waste. For instance, in the chemical industry, machine learning algorithms can adjust parameters in real-time to ensure optimal reaction conditions, thereby improving yield and reducing energy consumption.
Quality control is also benefiting from machine learning advancements. Traditional quality control methods often rely on manual inspections, which can be time-consuming and prone to human error. Machine learning algorithms, particularly those involving computer vision, can automate the inspection process, ensuring consistent and accurate quality assessments. This not only speeds up the production line but also enhances the overall quality of the products.
Furthermore, machine learning is playing a crucial role in energy management within industrial settings. By analyzing consumption patterns and predicting future energy needs, machine learning models can optimize energy usage, leading to cost savings and reduced environmental impact. This is particularly important in industries with high energy demands, such as manufacturing and mining.
Challenges in Implementing Machine Learning in Industrial Control Systems
Despite the promising applications, integrating machine learning into industrial control systems is not without its challenges. One of the primary obstacles is the quality and availability of data. Machine learning models require large volumes of high-quality data to function effectively. However, many industrial environments still rely on legacy systems that may not be equipped to collect or store the necessary data. This can hinder the development and deployment of machine learning solutions.
Another significant challenge is the complexity of industrial processes. Unlike consumer applications, where machine learning models can be trained on relatively straightforward tasks, industrial processes often involve intricate systems with numerous variables. Developing models that can accurately predict and control these processes requires a deep understanding of both machine learning techniques and the specific industrial domain.
Moreover, there is the issue of integration with existing systems. Many industrial facilities have invested heavily in traditional control systems, and transitioning to machine learning-based solutions can be costly and disruptive. Ensuring compatibility and seamless integration with existing infrastructure is crucial to the successful adoption of machine learning technologies.
Security is also a major concern. As industrial control systems become more connected and reliant on data, they become more vulnerable to cyber threats. Implementing robust security measures to protect sensitive data and ensure the integrity of machine learning models is essential to prevent potential disruptions and safeguard operations.
Finally, there is the challenge of workforce adaptation. The introduction of machine learning technologies requires a workforce that is skilled in both data science and industrial operations. Training and upskilling employees to work alongside these advanced technologies is vital to fully realize the benefits of machine learning in industrial control systems.
In conclusion, while machine learning offers significant advantages for industrial control systems, its implementation is accompanied by a set of challenges that must be addressed. By overcoming these obstacles, industries can harness the full potential of machine learning to drive innovation, efficiency, and competitiveness in the modern industrial landscape.