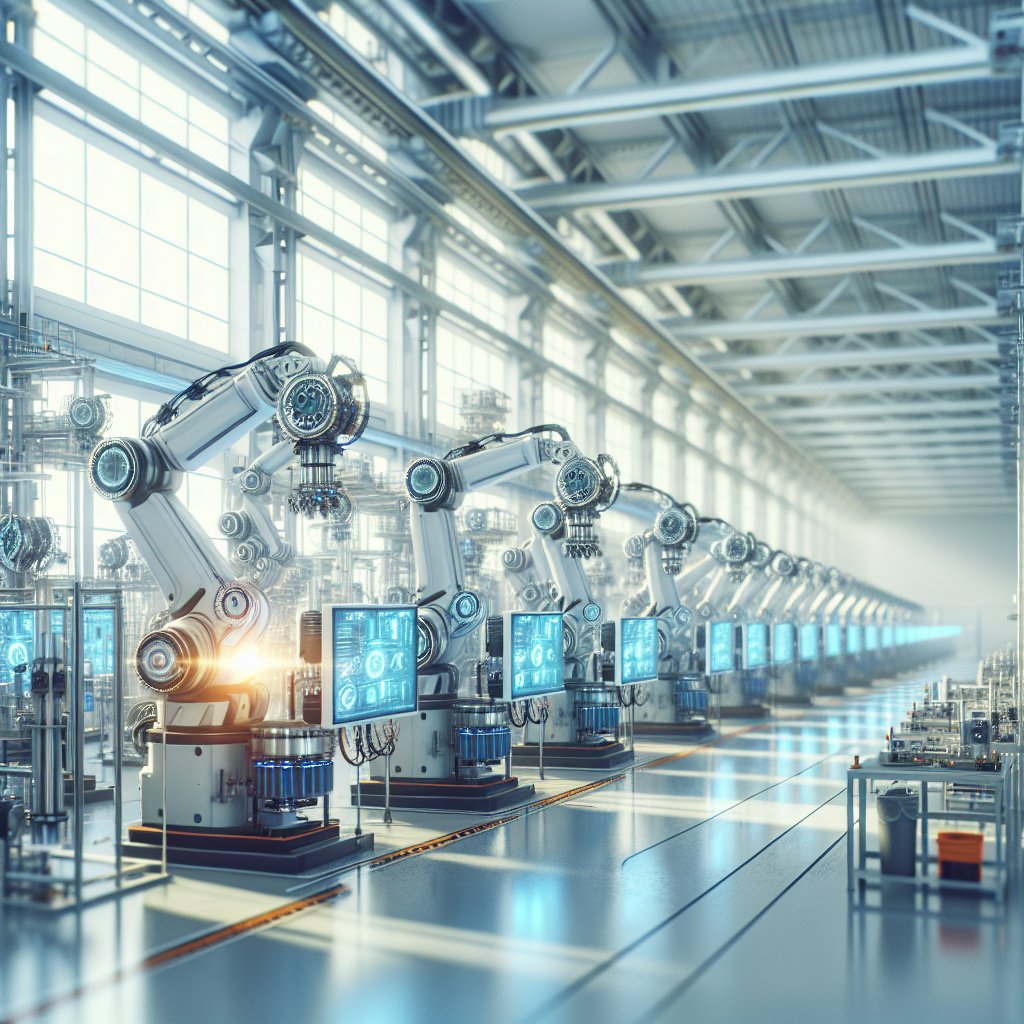
Machine learning is revolutionizing the way industries approach process optimization, offering unprecedented opportunities for efficiency and innovation. As industries strive to remain competitive in a rapidly evolving market, the integration of machine learning technologies into industrial processes has become a critical factor for success. This article explores the transformative impact of machine learning on industrial process optimization, highlighting key areas where these technologies are making a significant difference.
Understanding Machine Learning in Industrial Contexts
Machine learning, a subset of artificial intelligence, involves the use of algorithms and statistical models to enable computers to perform tasks without explicit instructions. In industrial contexts, machine learning is applied to analyze vast amounts of data generated by manufacturing processes, supply chains, and other operational activities. By identifying patterns and insights within this data, machine learning models can predict outcomes, optimize processes, and enhance decision-making.
One of the primary advantages of machine learning in industry is its ability to handle complex and dynamic systems. Traditional optimization methods often struggle with the variability and uncertainty inherent in industrial processes. Machine learning, however, excels in environments where data is abundant and conditions are constantly changing. This adaptability allows industries to fine-tune their operations in real-time, leading to improved efficiency and reduced costs.
Applications of Machine Learning in Process Optimization
Machine learning is being applied across various industrial sectors to optimize processes and drive innovation. Here are some key applications:
Predictive Maintenance
Predictive maintenance is one of the most impactful applications of machine learning in industry. By analyzing data from sensors and equipment, machine learning models can predict when a machine is likely to fail, allowing for timely maintenance and reducing downtime. This not only extends the lifespan of equipment but also minimizes disruptions to production schedules.
Quality Control
Ensuring product quality is a critical concern for manufacturers. Machine learning algorithms can analyze data from production lines to detect defects and anomalies in real-time. This enables manufacturers to address quality issues promptly, reducing waste and improving customer satisfaction.
Supply Chain Optimization
Machine learning is also transforming supply chain management by optimizing inventory levels, predicting demand, and improving logistics. By analyzing historical data and market trends, machine learning models can forecast demand more accurately, ensuring that the right products are available at the right time and place.
Energy Management
Industries are increasingly focused on reducing their energy consumption and carbon footprint. Machine learning can optimize energy usage by analyzing data from energy meters and production processes. This allows companies to identify inefficiencies and implement energy-saving measures, leading to cost savings and environmental benefits.
Challenges and Considerations
While the benefits of machine learning in industrial process optimization are significant, there are also challenges to consider. One of the primary challenges is the quality and availability of data. Machine learning models require large amounts of high-quality data to function effectively. In some cases, data may be incomplete, inconsistent, or siloed across different systems, making it difficult to leverage for machine learning applications.
Another consideration is the integration of machine learning into existing systems and workflows. Industries must ensure that their infrastructure can support the computational demands of machine learning models and that employees are trained to work with these new technologies. Additionally, there are concerns about data privacy and security, particularly when dealing with sensitive industrial information.
The Future of Machine Learning in Industry
The future of machine learning in industrial process optimization is promising, with ongoing advancements in technology and increasing adoption across sectors. As machine learning algorithms become more sophisticated and accessible, industries will continue to find new ways to leverage these tools for competitive advantage.
One area of future development is the integration of machine learning with other emerging technologies, such as the Internet of Things (IoT) and blockchain. By combining machine learning with IoT, industries can enhance data collection and analysis, leading to even more precise optimization. Blockchain, on the other hand, can provide secure and transparent data sharing, facilitating collaboration across supply chains.
Moreover, as machine learning models become more interpretable, industries will gain greater insights into the decision-making processes of these algorithms. This transparency will help build trust in machine learning applications and encourage wider adoption.
In conclusion, machine learning is transforming industrial process optimization by providing powerful tools for data analysis and decision-making. While challenges remain, the potential benefits are immense, offering industries the opportunity to enhance efficiency, reduce costs, and drive innovation. As technology continues to evolve, the role of machine learning in industry will only grow, shaping the future of manufacturing and beyond.