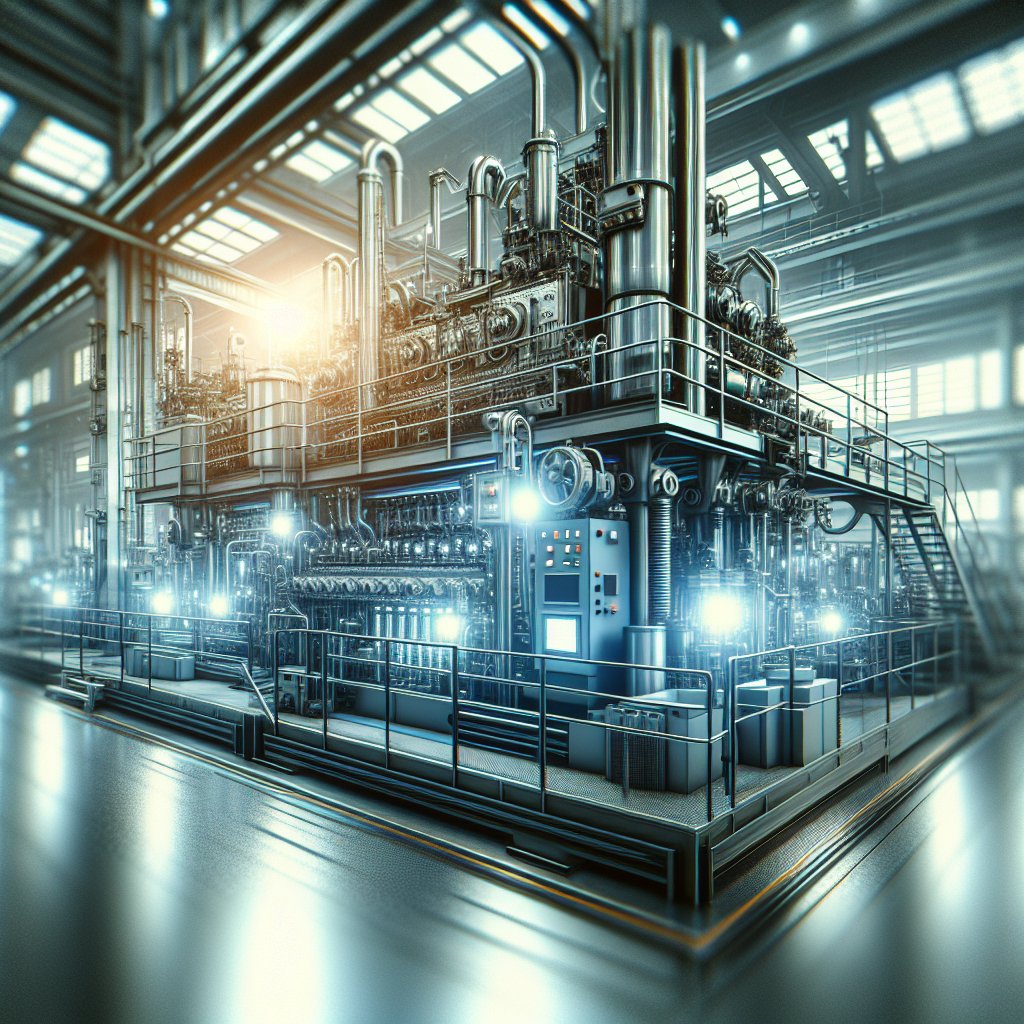
Industrial Machine Learning is revolutionizing the way industries approach predictive maintenance, offering unprecedented opportunities to enhance operational efficiency and reduce downtime. As industries continue to embrace digital transformation, the integration of machine learning technologies into maintenance strategies is becoming increasingly vital. This article delves into the transformative impact of machine learning on predictive maintenance, exploring its benefits, challenges, and future prospects.
The Role of Machine Learning in Predictive Maintenance
Predictive maintenance is a proactive approach that aims to predict when equipment failures might occur, allowing for timely interventions to prevent unexpected breakdowns. Traditionally, maintenance strategies have relied on scheduled maintenance or reactive maintenance, both of which have limitations. Scheduled maintenance can lead to unnecessary downtime and costs, while reactive maintenance often results in unexpected failures and costly repairs.
Machine learning, a subset of artificial intelligence, offers a more sophisticated approach by analyzing vast amounts of data to identify patterns and predict potential failures. By leveraging historical data, sensor readings, and other relevant information, machine learning algorithms can provide accurate predictions about equipment health and performance. This enables industries to optimize maintenance schedules, reduce downtime, and extend the lifespan of their assets.
Data Collection and Analysis
The foundation of machine learning in predictive maintenance lies in data collection and analysis. Modern industrial equipment is equipped with numerous sensors that continuously monitor various parameters such as temperature, vibration, pressure, and more. These sensors generate a wealth of data that can be harnessed to gain insights into equipment health.
Machine learning algorithms process this data to identify patterns and anomalies that may indicate potential issues. For instance, a sudden increase in vibration levels might suggest an impending mechanical failure. By analyzing these patterns, machine learning models can predict when maintenance should be performed, minimizing the risk of unexpected breakdowns.
Benefits of Machine Learning in Predictive Maintenance
The integration of machine learning into predictive maintenance offers several key benefits:
- Reduced Downtime: By accurately predicting when equipment is likely to fail, industries can schedule maintenance during planned downtime, minimizing disruptions to operations.
- Cost Savings: Predictive maintenance helps avoid costly emergency repairs and extends the lifespan of equipment, leading to significant cost savings over time.
- Improved Safety: Timely maintenance reduces the risk of equipment failures that could pose safety hazards to workers.
- Enhanced Efficiency: Optimized maintenance schedules ensure that equipment operates at peak efficiency, improving overall productivity.
Challenges and Considerations
While the benefits of machine learning in predictive maintenance are clear, there are several challenges and considerations that industries must address to fully leverage its potential.
Data Quality and Integration
One of the primary challenges is ensuring the quality and integration of data. Machine learning models rely on accurate and comprehensive data to make reliable predictions. Incomplete or inaccurate data can lead to incorrect predictions, undermining the effectiveness of predictive maintenance strategies.
Industries must invest in robust data collection and integration systems to ensure that data from various sources is accurate, consistent, and easily accessible. This may involve upgrading existing infrastructure, implementing new sensors, and adopting advanced data management solutions.
Model Training and Validation
Developing effective machine learning models requires careful training and validation. Models must be trained on historical data to learn patterns and relationships that indicate potential failures. However, this process can be complex and time-consuming, requiring expertise in data science and machine learning.
Additionally, models must be regularly validated and updated to ensure their accuracy and relevance. As equipment and operating conditions change, models may need to be retrained to account for new data and evolving patterns.
Scalability and Implementation
Implementing machine learning for predictive maintenance at scale can be challenging, particularly for large industrial operations with diverse equipment and complex processes. Industries must develop scalable solutions that can be seamlessly integrated into existing systems and processes.
This may involve collaborating with technology providers, investing in cloud-based solutions, and adopting standardized protocols for data exchange and communication. Scalability is crucial to ensure that predictive maintenance solutions can be effectively deployed across multiple sites and equipment types.
The Future of Predictive Maintenance with Machine Learning
As machine learning technologies continue to evolve, the future of predictive maintenance holds exciting possibilities. Emerging trends and advancements are poised to further enhance the capabilities and impact of predictive maintenance strategies.
Integration with IoT and Edge Computing
The integration of machine learning with the Internet of Things (IoT) and edge computing is set to revolutionize predictive maintenance. IoT devices enable real-time data collection and monitoring, while edge computing allows for data processing and analysis at the source, reducing latency and improving response times.
By combining these technologies, industries can achieve faster and more accurate predictions, enabling immediate interventions to prevent equipment failures. This integration also facilitates remote monitoring and management, allowing for more efficient maintenance operations.
Advanced Analytics and AI-Driven Insights
Advancements in analytics and artificial intelligence are driving the development of more sophisticated predictive maintenance solutions. Machine learning models are becoming increasingly capable of handling complex data sets and generating actionable insights.
AI-driven insights can provide industries with a deeper understanding of equipment performance, enabling more informed decision-making and strategic planning. This includes identifying root causes of failures, optimizing maintenance schedules, and predicting future maintenance needs with greater precision.
Collaboration and Industry Standards
Collaboration among industry stakeholders and the establishment of standardized protocols are essential for the widespread adoption of machine learning in predictive maintenance. Industry standards can facilitate data sharing, interoperability, and best practices, ensuring that predictive maintenance solutions are effective and reliable.
Collaboration between technology providers, equipment manufacturers, and industrial operators can drive innovation and accelerate the development of new solutions. By working together, stakeholders can address common challenges and unlock new opportunities for predictive maintenance.
In conclusion, industrial machine learning is unlocking new opportunities for predictive maintenance, transforming the way industries approach equipment management and maintenance. By harnessing the power of data and advanced analytics, industries can achieve greater efficiency, cost savings, and operational excellence. As technology continues to advance, the future of predictive maintenance promises even greater possibilities, paving the way for a more resilient and sustainable industrial landscape.